State of Generative AI in May 2024
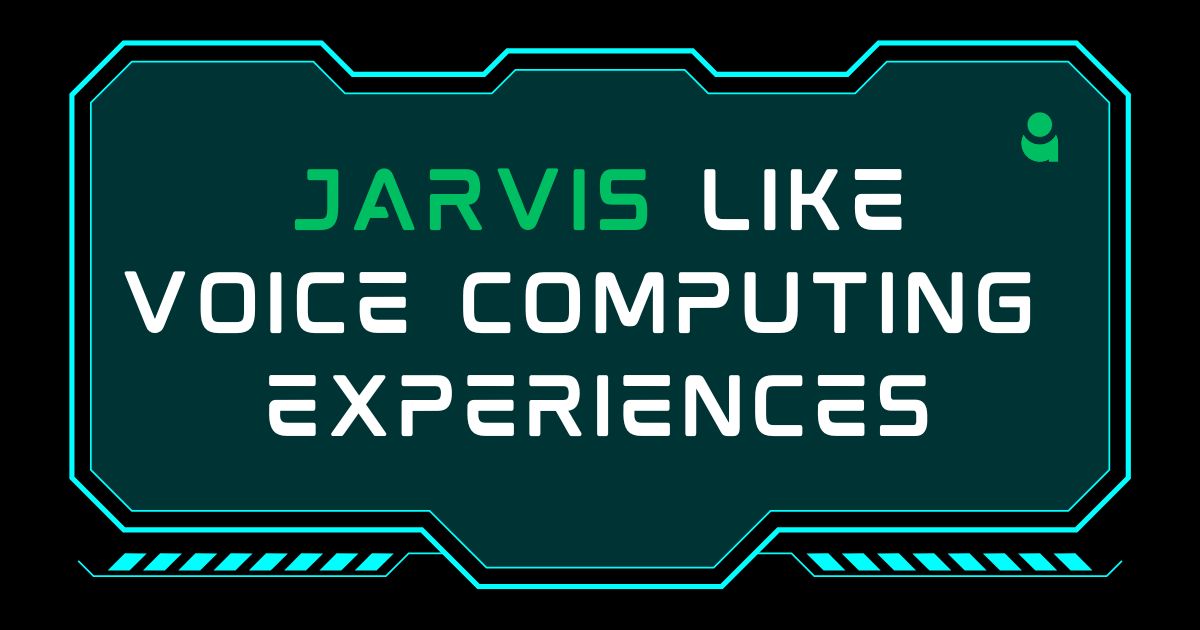
As a software engineer working with AI, I have been amazed by the rate at which Generative AI is progressing. Since the release of GPT-4 by OpenAI over a year ago, many have noted that the improvements in models have been incremental rather than a leap forward (think of what GPT-4 was to GPT-3.5) in terms of their benchmarks. People speculate that we may be heading towards diminishing performance returns using today’s architecture. They should be cautious though, as there may be major announcements from vendors releasing new versions of their models (think GPT-5 from OpenAI) soon(ish). This blog, however, only looks at publicly available large language models (LLMs) and multimodal models today like GPT-4o, Gemini 1.5Pro, Pi, Claude Opus, Llama-3, Mixtral, etc as of May 2024.
I use many of these AI technologies in my day-to-day work. For instance, I use GPT-4o for drafting documents, emails, and code, Perplexity for diving deep into topics of interest, and Pi for authentic, natural conversations. There is no doubt that these AI technologies and others have already changed our world. According to GitHub, their Co-Pilot product makes developers more productive by up to 55%. Goldman Sachs estimates that Generative AI could raise the global GDP by around $7 trillion USD over the next decade. Most people who have used these AI technologies would probably agree that it has helped them be more productive, whether it be completing assignments, building features, drafting proposals, brainstorming, or any other text-based creative endeavor.
Like most people, I was fascinated by ChatGPT when it came out. While it was still rough around the edges with issues like hallucinations, it worked like a charm for most of my queries. It still felt like interacting with a computer: it was text-based, responses were not instant, and it was prone to hallucinations. Fast forward 18 months, and all of that changed for me. Last week, the world saw two major Generative AI announcements from Google and OpenAI that led me to rethink Generative AI and what it could be. I am referring to the announcement of GPT-4o by OpenAI and Project Astra by Google.
Multimodal Models
Both GPT-4o and Project Astra (powered by Gemini) are currently not available to the public. They were announced last week, and we saw some live demos showing what these models were capable of. These models were built from the ground up to be multimodal, which means they can understand and operate with different types of data like text, code, audio, images, and video. While we have had multimodal models for a while now, these two announcements were quietly groundbreaking.
I frequently use ChatGPT’s voice mode (not GPT-4o) on my iPhone to quench my curiosity every now and then. Using voice mode to interact with the models has not been a great user experience. There are quite a few problems, like ChatGPT speaking over the user and taking a couple of seconds to respond to even simple queries. While this is already a wonderful feature to be able to interact with AI using your voice and get natural-sounding responses, the UX problems I mentioned above still make it feel like interacting with a computer.
Sub-400 Milliseconds Voice Latency Makes a Difference
Today, ChatGPT voice mode has a latency of 3-5 seconds before it starts to respond. The announcement from OpenAI claims GPT-4o has voice latency of less than 400 milliseconds. According to OpenAI, GPT-4o (“o” for “omni”) is a step towards much more natural human-computer interaction. It can respond to audio inputs in as little as 232 milliseconds, with an average of 320 milliseconds, which is similar to human response time in a conversation. Because these models are multimodal, they can natively process voice, observe tone, and notice background audio, etc.
This new capability is profound and has huge opportunities. Sam Altman, CEO of OpenAI, stated on his blog, “Talking to a computer has never felt really natural for me; now it does.” I have not personally used the new voice mode, so my thoughts are based on the demo I saw. The new sub-400 milliseconds voice response is probably the best user interface I have seen. The demos almost make it seem like speaking with the technology from the 2013 movie “Her.” It is natural, expressive, instant, and human-like. It also handles interruptions quite well, so you can start speaking while the AI is speaking. Check out this demo to see what I mean.
These models can also natively process images and video, which I will not discuss in this post.
Environmental Concerns
Training and running inference on these massive Large Language Models and Multimodal Models is frighteningly energy-intensive. The amount of electricity used to train GPT-4, for example, would be enough to power 1,300 US homes for a year. This is approximately 7 million kilograms of CO2 emissions. That is just the training cost; the inference costs can easily surpass that. Historically, with Google, the balance was 60 percent inference, 40 percent training. If Google were to replace all of their ~9 billion searches a day with Generative AI, Google would need as much power as Ireland just to run its search engine. It is clear that Generative AI is already having a huge impact on the environment.
Conclusion
While many argue that the rate of advancement of Generative AI may be plateauing, I believe the new sub-400 millisecond latency voice conversations enabled by the latest multimodal models are a step up from convention. The ability to speak with a computer, as seen in movies like “Iron Man” and “Her,” is profound. The models are also learning new languages and becoming more capable across the board. It is indeed an exciting time to be alive and to be experimenting with AI technology.
AI technologies come with many risks, such as misinformation, bias, hallucinations, regulatory challenges, etc. It is also important to note the environmental impact that training and running inference over these models has is alarming. While another hyped-up technology, blockchain, may be a worse offender in environmental terms, the impact of Generative AI is far from trivial. As vendors build bigger models using the current architecture, the environmental impact is likely to worsen. The challenge will be striking a balance between creating incredible user experiences and reducing the impact on the environment.